Harnessing the Power of Medical Datasets for Machine Learning
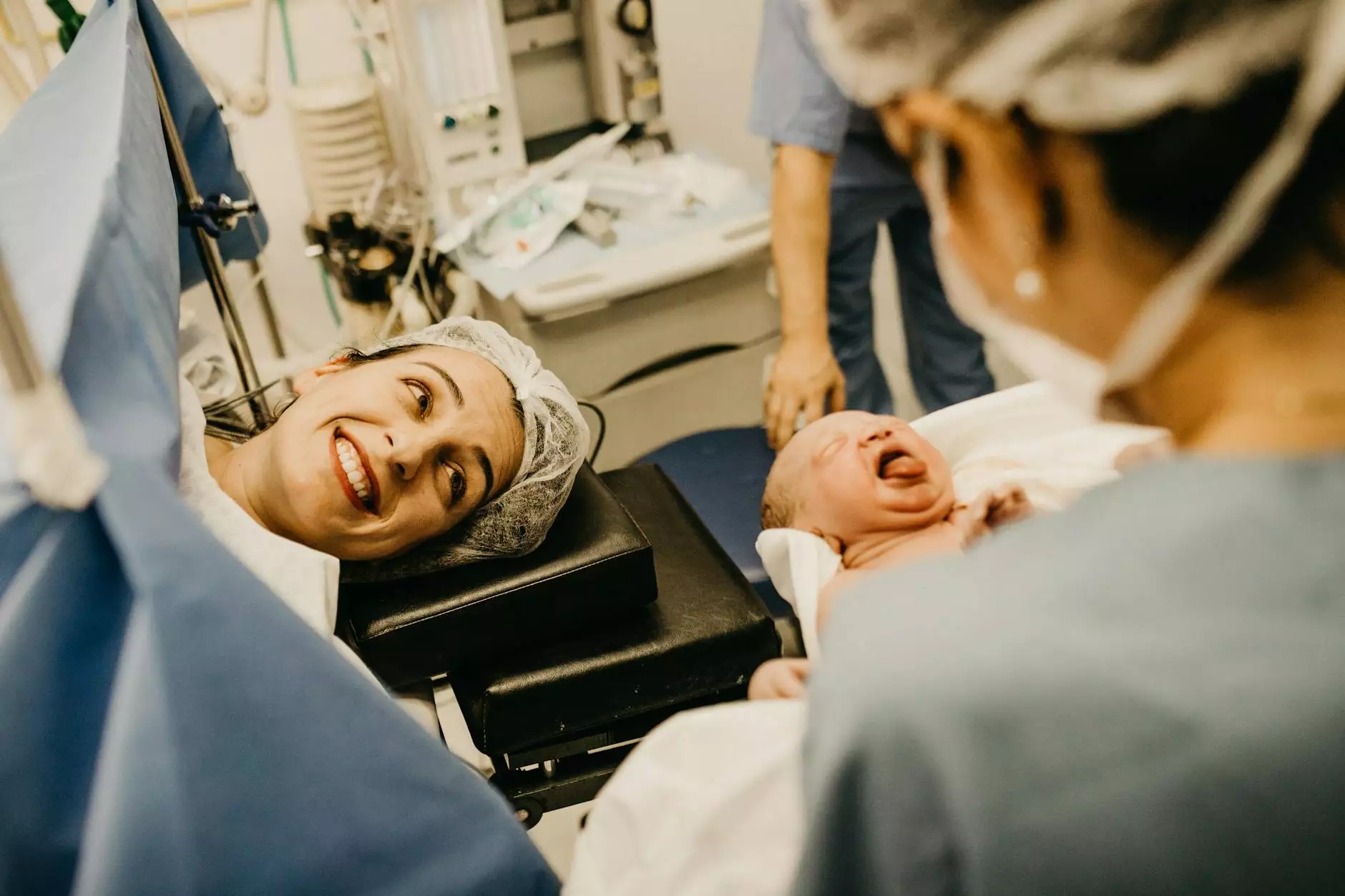
In an era where technology is seamlessly integrating into various sectors, healthcare stands out as one of the most benefited industries from advancements in machine learning. At the core of these advancements are medical datasets, which serve as the backbone for training machine learning algorithms to enhance patient care, streamline processes, and introduce innovative solutions. This article delves into the significance of medical datasets for machine learning and how they are reshaping the future of healthcare.
Understanding Medical Datasets
Medical datasets encompass a wide range of information collected from various healthcare settings. These datasets can include patient demographics, medical histories, diagnostic data, treatment outcomes, and even genetic information. The goal of compiling such rich data is to extract actionable insights that can help improve patient outcomes. Let's explore the different types of data commonly found in these datasets:
Types of Medical Datasets
- Electronic Health Records (EHRs): Comprehensive records of patient health information maintained by healthcare providers.
- Clinical Trial Data: Data derived from clinical studies assessing the safety and effectiveness of new treatments.
- Genomic Data: Information related to genes and their functions, which is crucial for personalized medicine.
- Radiology Images: Medical imaging data that can be analyzed using machine learning techniques for diagnosis.
- Wearable Device Data: Real-time health tracking data collected through devices such as smartwatches and fitness trackers.
The Role of Machine Learning in Healthcare
Machine learning is a subset of artificial intelligence that enables systems to learn from data, identify patterns, and make predictions without explicit programming. Its application in healthcare is profound, offering unparalleled opportunities to enhance disease diagnosis, treatment customization, and operational efficiencies. With the influx of medical datasets, machine learning's role in healthcare is expanding exponentially.
Key Applications of Machine Learning in Healthcare
- Predictive Analytics: Leveraging historical data to predict patient outcomes and potential health risks.
- Image Recognition: Analyzing diagnostic images to assist radiologists in identifying abnormalities.
- Natural Language Processing: Extracting meaningful insights from unstructured data, such as clinical notes.
- Treatment Recommendations: Using data-driven approaches to suggest personalized treatment plans.
- Operational Optimization: Enhancing administrative processes and resource allocation within healthcare facilities.
Advantages of Using Medical Datasets for Machine Learning
The integration of medical datasets with machine learning techniques offers numerous benefits that can transform the healthcare landscape.
1. Enhanced Diagnostic Accuracy
Machine learning algorithms can analyze vast amounts of medical data to identify patterns that may be missed by human clinicians. By improving diagnostic accuracy, healthcare providers can ensure timely treatment interventions, ultimately leading to better patient outcomes.
2. Personalized Medicine
With access to genetic and genomic data, machine learning enables the development of tailored treatment plans that cater to individual patient profiles. This approach enhances the efficacy of treatments and minimizes adverse effects.
3. Cost Efficiency
By optimizing operational processes and reducing unnecessary tests and procedures, machine learning applications can significantly lower healthcare costs. Efficient resource allocation ensures that patients receive care when they need it most.
4. Accelerated Drug Development
Machine learning models can streamline the drug discovery process by predicting how different compounds will interact within biological systems. This capability can lead to faster development and deployment of new therapies.
5. Disease Surveillance and Population Health
By analyzing trends in health data, machine learning can assist public health officials in tracking disease outbreaks and assessing the overall health of populations. This proactive approach enhances preparedness and response strategies.
Challenges in Utilizing Medical Datasets
Despite the significant advantages, the use of medical datasets for machine learning is not without challenges.
1. Data Privacy and Security
Given the sensitive nature of health information, compliance with regulations such as HIPAA in the U.S. is critical. Maintaining patient confidentiality while leveraging data is a considerable challenge that must be addressed proactively.
2. Data Quality and Integration
Inconsistencies, missing values, and variations in data collection methods can compromise the quality of medical datasets. Ensuring data accuracy and developing standardized protocols for data integration is vital for meaningful analysis.
3. Algorithm Bias
Machine learning models are only as good as the data they are trained on. If medical datasets are not diverse or comprehensive, the resulting algorithms may reflect biases, leading to inequitable healthcare outcomes.
Future Trends in Medical Datasets and Machine Learning
The future of medical datasets in conjunction with machine learning appears promising, with several trends poised to transform the healthcare industry further.
1. Increased Interoperability
As healthcare systems strive for better integration, the ability for different data systems to communicate will enhance data sharing and improve patient care across various platforms.
2. Real-Time Data Analysis
The rise of wearable technology and remote monitoring tools promises real-time health data collection, allowing for immediate analysis and intervention when necessary.
3. Advanced Data Ethics
As the utilization of medical datasets grows, so does the focus on ethical considerations. Establishing guidelines that govern data use while prioritizing patient rights and trust will be essential.
4. Integration of Artificial Intelligence
The fusion of machine learning with other AI technologies will catalyze new applications in healthcare, taking large-scale data analysis to new heights and enabling sophisticated predictive models.
Conclusion
The intersection of medical datasets and machine learning is a groundbreaking frontier in healthcare innovation. With their ability to streamline processes, personalize patient care, and enhance diagnostic accuracy, these medical datasets serve as invaluable resources that healthcare providers can harness to meet contemporary challenges. Despite existing obstacles, the potential for improved patient outcomes is immense, paving the way for a future where technology and healthcare evolve together. As we continue to explore this dynamic synergy, it is crucial to remain vigilant regarding ethical practices and data integrity to ensure that the benefits of machine learning are realized for all.
In conclusion, the integration of medical datasets for machine learning is not just a trend; it's a transformative approach that is set to redefine the healthcare landscape, benefiting both practitioners and patients alike.
medical dataset for machine learning